Jibo Snap
World’s first robot photographer in the home
> UX Design
> Rapid Prototyping
> Motion Design
> Usability Testing
Skills Focus
01 —
Situation
One of Jibo’s first selling points was his ability to take pictures. This was a promise that must be paid off in a skill expected to see daily use. The tools in Jibo’s unique toolbox included natural language conversational UI, computer vision especially for detecting faces, and sound localization. Using these elements, the task was to create a photo capture skill only Jibo could do!
Hey Jibo, Take A Picture!
< < <
Hey Jibo, Take A Picture! < < <
— 02 —
Approach
A phrase we often used was “Jibo is more than a tablet on a stick.” He has a personality; he’s creative and curious. This element of his narrative dictated that Jibo should be a less of the camera and more the photographer. Making this happen meant including Jibo as a persona in the design process from the start. By prototyping early at different fidelities, and testing as often as possible, we found ways to reduce friction and let Jibo’s voice shine through.
— 03
Result
When Jibo was released, the Snap skill emerged as the second most used feature, resonating especially well with children. The positive reception underscores the seamless integration of technology into daily life, where the robot's charming personality garnered widespread appreciation from crowd-funding backers and early adopters. The feature's popularity, despite challenges with picture quality, helped to validate the role personality plays in enhancing the user’s experience and sentiment and landing Jibo on the cover of Time Magazine.
Rapid Prototyping
It almost sounds crazy in retrospect, but our very first prototype was that I just sat in the company kitchen and told people I could take a picture of them. I wanted to know what the challenges of a stationary photographer would be. What could they see? When would they get a good photo? How would the models react? Even just this simple move of acting like Jibo taught us a lot about the challenges we’d be up against.
Our First Nemesis: Lighting!
Something that became clear from my simple experience of stationary photography was that Jibo would have almost no control over the light. This would be especially challenging because, in addition to the quality of photos, it would affect Jibo’s ability to find faces. Discovering this limitation early led us to a greater emphasis on sound localization and improving prompts to solve for issues with facial recognition on first user request.
As soon as we could run a simplified version of the experience on Robot we ramped up user testing. There were some disagreements between UX and the narrative team about displaying a viewfinder on Jibo’s face. The concern from a narrative perspective was that showing a viewfinder would make Jibo seem more like a tool than a personality. However, data showed that displaying the viewfinder was encouraging people to stand still at the point of photo capture and ultimately increased the number of photos kept. After prototyping several options, we ultimate landed on a tiered solution. If the skill was going well, Jibo found a face and oriented quickly, we would show a corner frame animation, then only show the viewfinder for a very short time right before photo capture. This way more of the experience held the emotional impact the narrative team hoped for while keeping the positive usability outcomes.
Early exploration to optimize the flow
Conversation Tree
Jibo Snap is triggered with a simple command to take a picture or equivalent. From there, the bulk of the process is handled by sound localization and computer vision. The goal of the happy path is to have Jibo do the heavy lifting. If he can find a face quickly and frame the picture he will just take it (as shown in the videos below). If the process is not as clean, he will be more talkative and provide more feedback to the user. As Adam Savage would say, “Failure is always an option,” so he has to fail gracefully when it happens. If he is unable to find a face after 60 seconds of searching while giving additional prompts he will simply show you what he is looking at and ask if you want to take that photo. This allows one last chance for users to align themselves in the photo if Jibo got close enough without knowing it.
Final Interaction
In the final interaction, we included a few extra pieces of user feedback to be as clear as possible how confident Jibo was in taking the photo. In most cases he is very confident, finds people immediately and snaps the photo. If he isn’t as confident, our narrative team wrote prompts that properly implied there were challenges. Beta testing with the robot in people’s homes showed this skill was very popular among children, so tweaking was done to make sure Jibo would check lower to the ground and give kid friendly instructions to get the best photos.
Credit to Heather Mendonça and Kim Hui for the original camera illustration and animation respectively. I adjusted the original animation and added elements to account for behavior found in user testing.
Learning and Growth
This was my first time working on a conversational interface and leveraging computer vision to capture user input. To get this exactly right lead developer, Bard McKinley, and I spent hours role playing as different users. We ran more user tests on this feature than any other launch skill to make sure that Jibo appeared smart to our end users.
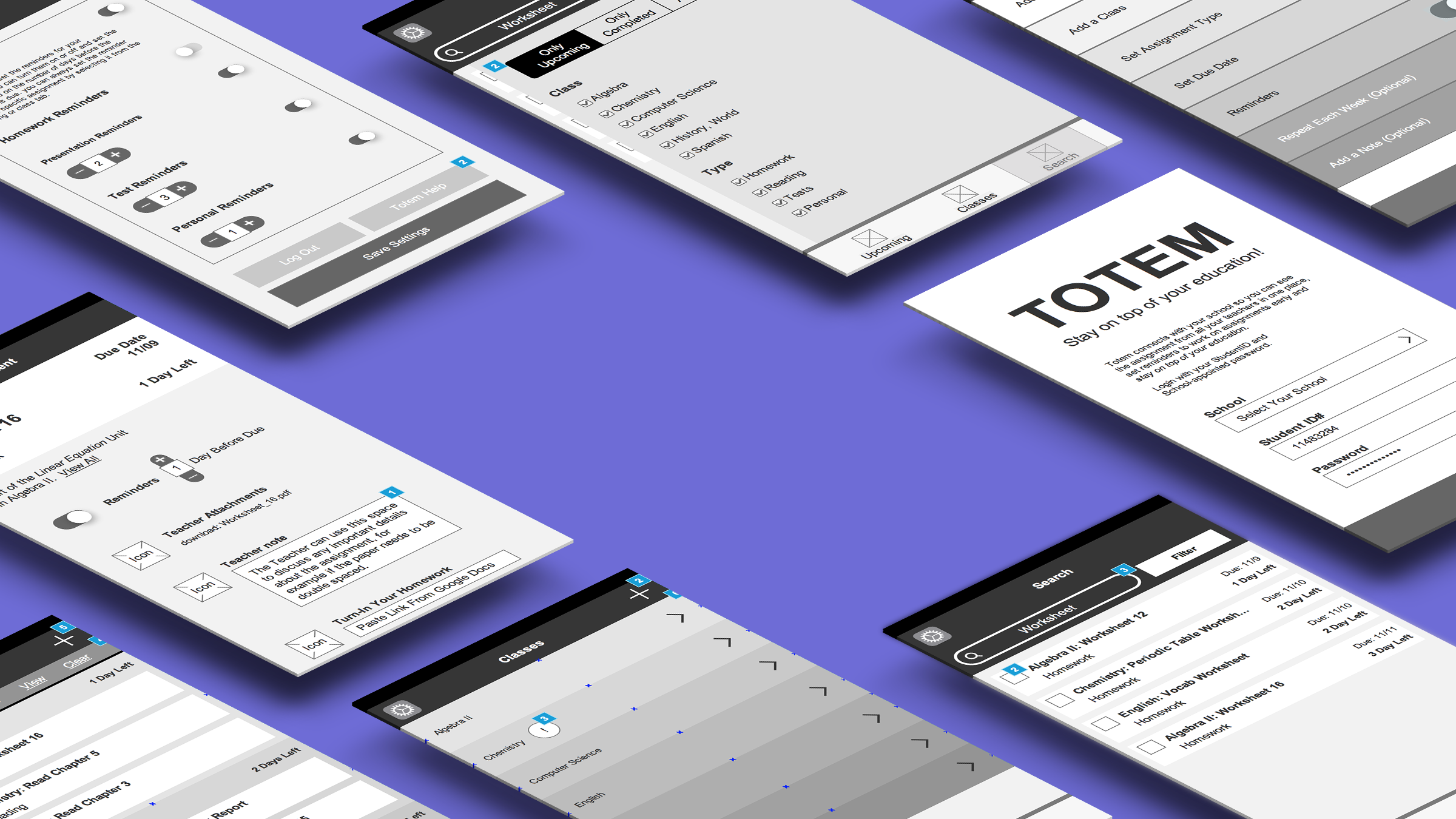